[paper-review] Reactive Base Control for On-The-Move Mobile Manipulation in Dynamic Environments
RA-L. [Paper] [Project Page]
Ben Burgess-Limerick1, 2, Jesse Haviland1, 2, Chris Lehnert1, Peter Corke1 1Queensland University of Technology Centre for Robotics (QCR), Brisbane, Australia 2 CSIRO Data61, Brisbane, Australia
Mar. 03
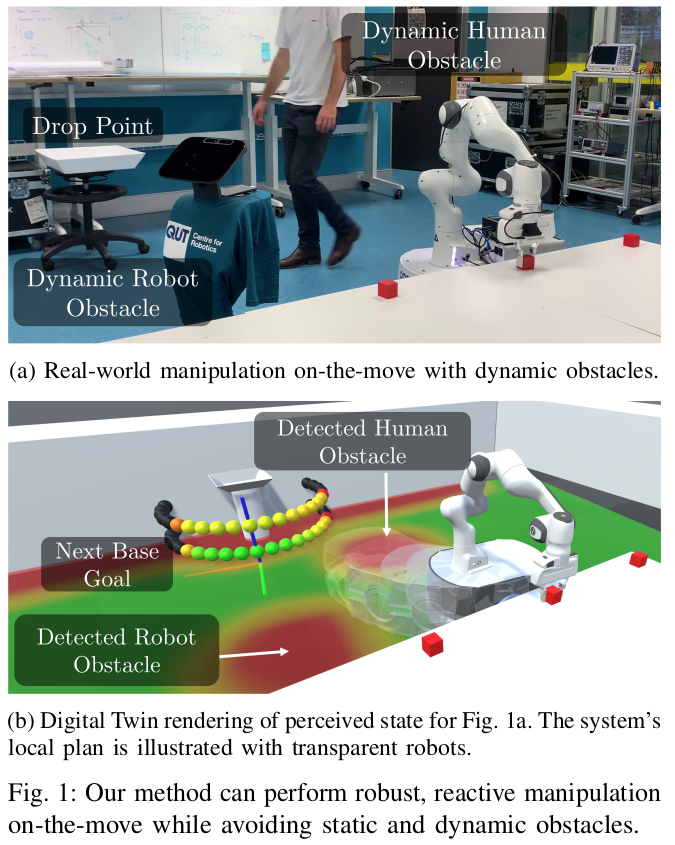
Fig. 1: Overview of Reactive Base Control.
Summary
- This paper presents a reactive-based control method for mobile manipulation in dynamic environments, which significantly reduces the task time and improves the performance of the robot while avoiding static and dynamic obstacles.
- This approach contrasts with traditional methods where the base and manipulator are controlled separately, often resulting in increased task time due to the sequential completion of navigation and manipulation tasks.
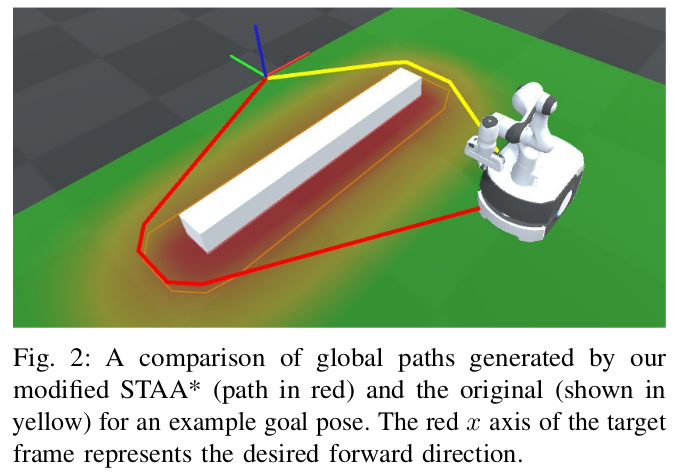
Fig. 2: Inclusion of orientation cost in STAA*.
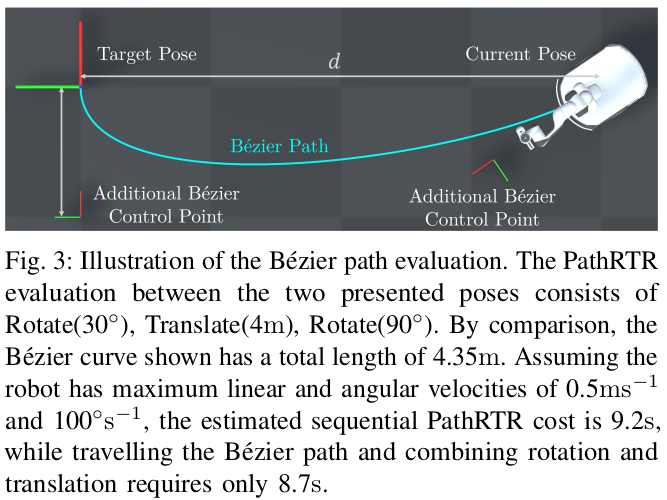
Fig. 3: Bezier path evaluation with PathRTR.
Contribution
- The paper introduces a reactive base control system for mobile manipulation in dynamic environments, enabling tasks to be performed on-the-move and significantly reducing total task time. This work marks the first real-world demonstration of reactive manipulation with both static and dynamic obstacles, showcasing the method’s practical applicability and robustness.
- 1) They used STAA* algorithm that plangs a collision-free global path by searching through a visibility graph, and then compuites the intersection of the global path and the borader of a local planning region to develop an intermediate goal. The most important addtion to STAA* is the inclusion of an orientation to the goal state. They consider orientation which enables poses to be achieved that smoothly connects the immediate target with the next goal.
- 2) In A* graph search module, they employed PathRTR heuristic. And they combines it with an additional heuristic based on a Bezier curve. It encourages the exploration of states that can be connected to the goal through smooth curves. They said that a value of 25% results in curves that work well in experimental setup.
- 3) Base Placement was optimized by simple cost functions by the weighted sum of two components:
- \[C_i = C_{i,C} + 1.05 \cdot C_{i,N}\]
- \(C_{i,C}\) is the estimated cost from robot to candidate.
- \(C_{i,N}\) is the estimated cost from the candidate to the next target.
- The path cost for each candidate is evaluated using the PathRTR metric.
- \[C_i = C_{i,C} + 1.05 \cdot C_{i,N}\]
- 4) Arm Obstacle Avoidance: They leveraged Quadratic Program to calculate joint veloicited for a given desired end-effector and base velocity. The controller allows for slack in the achieved end-effector velocity.
Thought
- I was already aware of a previous paper by this author, Manipulation On-The-Move, and it seems to be a follow-up to both this paper and an algorithm called STAA*.
- I think the contribution of this paper is to add a couple of heuristics to the existing algorithm, and I would have to start with the previous paper; the baseline controller implemented in this paper is all based on the previous paper as a reference.
- STAA* / MotM / PathRTR
- After reading the paper and watching the demo video, I was quite impressed with the holistic approach to motion planning for mobile-manipulator systems; so accurate and high performance.
Enjoy Reading This Article?
Here are some more articles you might like to read next: