[paper-review] Text2Reaction : Enabling Reactive Task Planning Using Large Language Models
RA-L, 2024. [Paper]
Zejun Yang , Li Ning, Haitao Wang, Tianyu Jiang, Shaolin Zhang, Shaowei Cui, Hao Jiang, Chunpeng Li, Shuo Wang and Zhaoqi Wang
May. 05.
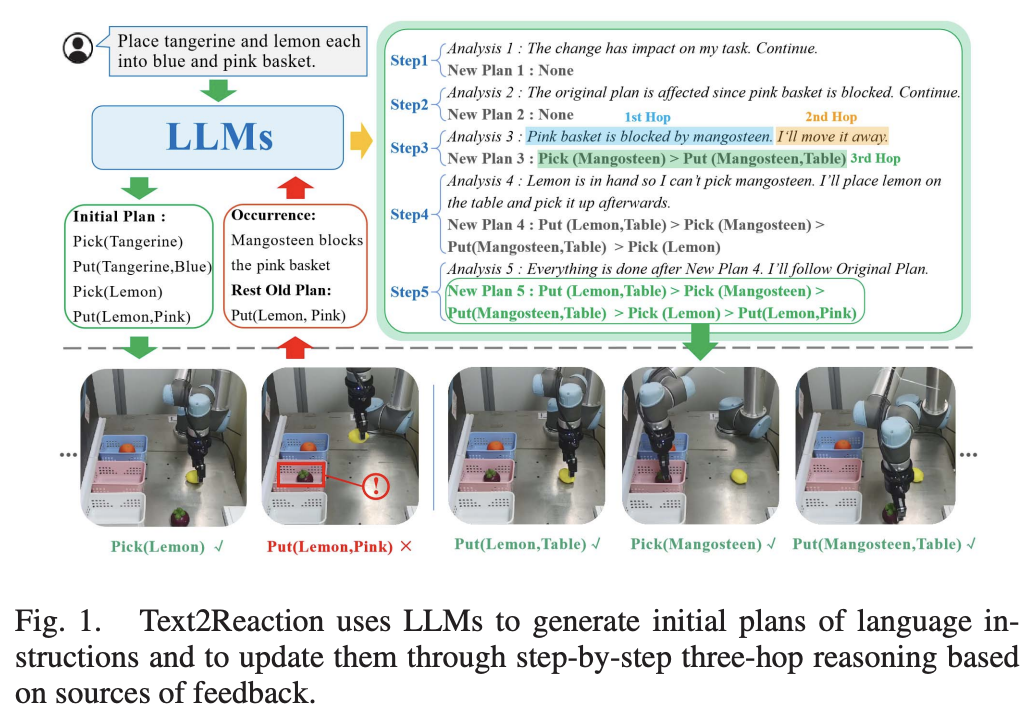
Fig. 1: Overview of Text2React.
Title:
Text2Reaction : Enabling Reactive Task Planning Using Large Language Models (R-AL, 2024)
Summary:
They propose Text2Reaction, an LLM-based framework enabling robots to continuously reason and update plans according to the latest environment changes.
Contribution:
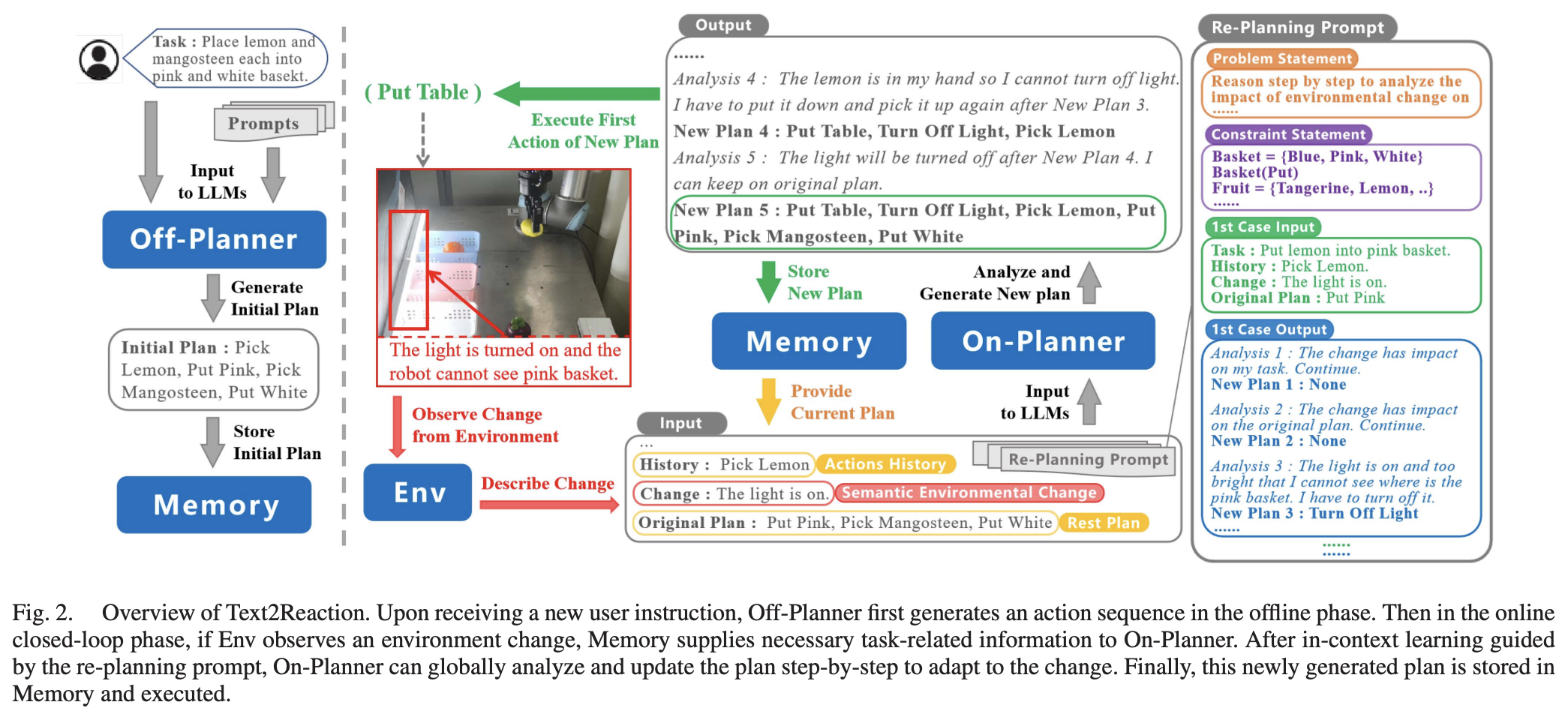
Fig. 2: Flowchart of Text2React.
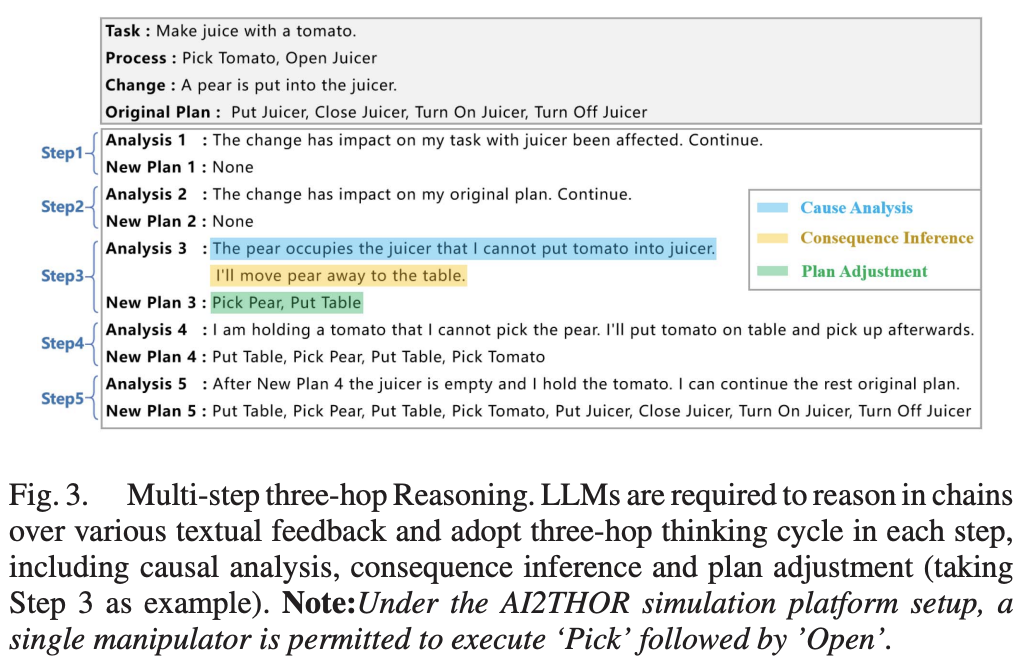
Fig. 3: Reasoning Steps of Text2React.
- They present the Re-planning Prompt, which informs LLMs the basic principles of re-planning.
- It fosters the gradual development of a current plan to a new one in a three-hop reasoning manner: cause analysis, consequence inference, and plan adjustment
- OffPlanner: an LLM-based planner that generates initial plans
- On-Planner: another planner, which updates plans under the guidance of the re-planning prompts
Thoughts:
- Re-planning is an important part of the reactive robot.
- They showed an LLM-based framework capable of comprehensively analyzing various feedback and continuously re-planning in response to environment changes.
- They propose new evaluation metrics for the success rate of task replanning: Executability Rate(ER), Success weighted by Path Length(SPL).
Enjoy Reading This Article?
Here are some more articles you might like to read next: